CNC Machining Industry trends
CNC Machining Industry: new & important trend
By: CapableMaching
Preface:
CNC machining is an important part of manufacturing that uses computer-controlled machines to process metals, plastics, and other materials. With the continuous development of technology, the CNC machining industry is undergoing major changes.
This article will introduce two major trends in the CNC machining industry: hybrid manufacturing and artificial intelligence algorithm application. These trends will have a profound impact on the manufacturing industry and bring new opportunities and challenges to enterprises.
CNC manufacturing history traces back to the 1940s, evolving from manually controlled machines to early computer-controlled systems in the mid-20th century. These early systems were revolutionary, paving the way for the sophisticated CNC machines we have today.
In recent years, CNC manufacturing has advanced a lot. New CNC machines use high-tech features like AI algorithms, special sensors, and real-time tracking. These upgrades make the process more accurate, improve production, and allow for flexible manufacturing.
Also, a new trend called hybrid manufacturing is gaining popularity. It blends a 3D printer with a CNC machine. This mix combines CNC machining precision with 3D printing’s ability to create complex designs quickly.
This article will discuss in detail the two main trends in the CNC machining industry. They are hybridization, AI algorithm applications, and automation in small-volume productions.
Hybrid Additive-Subtractive Manufacturing
The Future of Synergistic Production
Introduction
Hybrid Additive-Subtractive Manufacturing (HASM) is a blend of two powerful manufacturing methods: adding material in layers and cutting away excess. It’s like sculpting and building at the same time. This modern approach is making waves in manufacturing by allowing precise, efficient, and intricate production.
In this article, we’ll dive into HASM, explaining its basics, why it’s important, where it’s being used, and how it’s shaping the future of manufacturing. We’ll also touch on the technology involved, how to plan the process, what machines are available, and the challenges this method faces. Understanding HASM opens up a world of advanced manufacturing and exciting possibilities.
What is hybrid additive-subtractive manufacturing?
To understand hybrid additive-subtractive manufacturing (HASM), let’s break down its components: additive manufacturing and subtractive manufacturing.
- Additive Manufacturing: Additive manufacturing involves building an object by adding material layer by layer. It’s akin to how a 3D printer creates an object by depositing material one slice at a time, eventually forming a complete item. Each layer stacks on top of the previous one, following a digital design.
- Subtractive Manufacturing: On the other hand, subtractive manufacturing is like carving a sculpture from a block of wood. It starts with a larger piece and involves removing material to shape and refine it. For example, a CNC machine carves intricate patterns from a solid block of material.
Hybrid Additive-Subtractive Manufacturing (HASM): HASM is where these two techniques come together. It starts by adding material, layer upon layer, to form a base. Then, it employs subtractive methods to precisely shape and fine-tune the product. It combines the strengths of both techniques, enabling a more efficient and detailed production process. It’s like crafting a sculpture by adding layers and then carving intricate details into it to achieve a masterpiece.

Why Hybrid Additive-Subtractive Manufacturing Emerged
Hybrid Additive-Subtractive Manufacturing (HASM) has evolved as a response to the challenges and opportunities posed by additive manufacturing (AM) and subtractive manufacturing methods.
- Leveraging Additive Manufacturing: Additive manufacturing is excellent for creating intricate and complex parts that are difficult to produce using traditional methods. It involves layering materials to construct a 3D object. However, AM often falls short in achieving precise dimensions, geometric accuracy, and high surface quality compared to traditional manufacturing.
- Tapping into Subtractive Manufacturing: On the other hand, subtractive manufacturing, a traditional method, achieves high precision but may generate material waste during the cutting and shaping process. It involves starting with a block of material and then removing unwanted sections to obtain the desired shape.
- Merging the Best of Both Worlds: HASM ingeniously blends additive and subtractive approaches. It begins with additive manufacturing to build the primary structure, utilizing its advantages in creating intricate and complex geometries. Then, it applies subtractive techniques to refine the structure, enhancing surface smoothness and achieving precise geometry and dimensions.
- Addressing Additive Manufacturing Limitations: Additive manufacturing alone often falls short in surface quality and precision, especially for critical applications like aerospace, where precise tolerances are essential. HASM steps in to address these limitations by incorporating subtractive processes to enhance the end product’s final characteristics.
By combining the strengths of both additive and subtractive manufacturing, HASM offers a solution that brings efficient, precise, and detailed production within reach. It optimizes material usage, reduces waste, and significantly advances the realm of modern manufacturing, catering to the needs of industries where intricate, high-precision components are paramount.
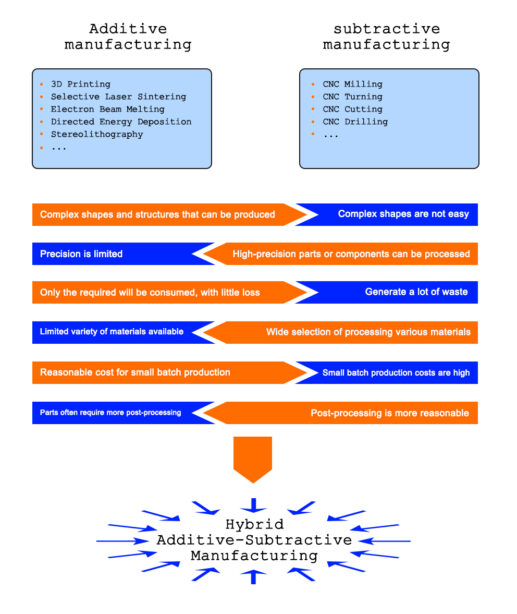
Advantages of Hybrid Additive-Subtractive Manufacturing
Hybrid Additive-Subtractive Manufacturing (HASM) offers a multitude of advantages that position it as a promising manufacturing approach for a wide array of applications.
- Enhanced Precision and Surface Quality: By combining additive and subtractive processes, HASM achieves superior precision and surface quality compared to traditional additive manufacturing. The subtractive stage allows for meticulous refinement, resulting in parts with exceptionally smooth surfaces and precise dimensions.
- Optimal Material Utilization: HASM optimizes material usage, with material waste minimized through the additive phase. This reduction in waste is particularly significant when dealing with expensive or specialized materials, making HASM a sustainable and cost-effective choice.
- Efficient Complex Geometries: The additive aspect of HASM excels in creating complex and intricate geometries that would be challenging or impractical using purely subtractive methods. This is especially beneficial for industries where complex part structures are a necessity.
- Integrated Process Flow: HASM integrates additive and subtractive steps into a single seamless process, saving time and resources by eliminating the need for multiple setups. This streamlined workflow increases efficiency and reduces production lead times.
- Energy-Efficient and Environmentally Friendly: The combination of additive and subtractive processes into one machine reduces energy consumption compared to operating separate machines for each process. This amalgamation aligns with sustainability objectives, making HASM an environmentally friendly choice.
- Broad Applicability: HASM’s adaptability lends itself well to a diverse range of industries, including aerospace, automotive, medical, and more. Its ability to cater to various sectors underlines its versatility and potential to revolutionize modern manufacturing.
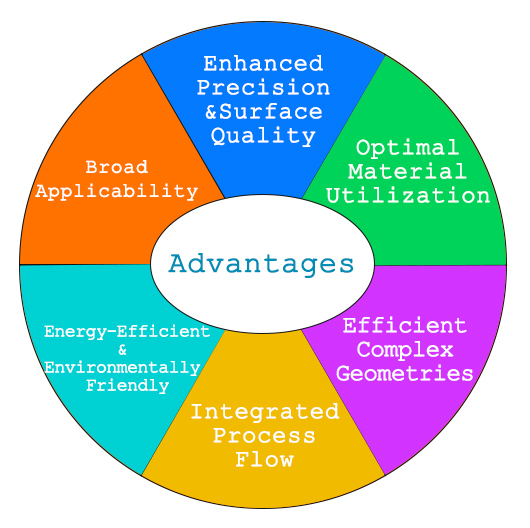
Research Status of Hybrid Additive-Subtractive Manufacturing Based on Different Energy Sources
- Hybrid Manufacturing Utilizing Electric Arc as an Energy Source
The combination of electric arc melting and milling stands as a common and prevalent approach in hybrid additive-subtractive manufacturing. This method involves depositing a layer of material by melting filaments using the high temperature generated by an electric arc and then precisely milling the deposited layer to achieve a smooth surface. Through this repetitive process, high-precision components are fabricated. The most common arc-based 3D printing is WAAM (Wire Arc Additive Manufacturing).
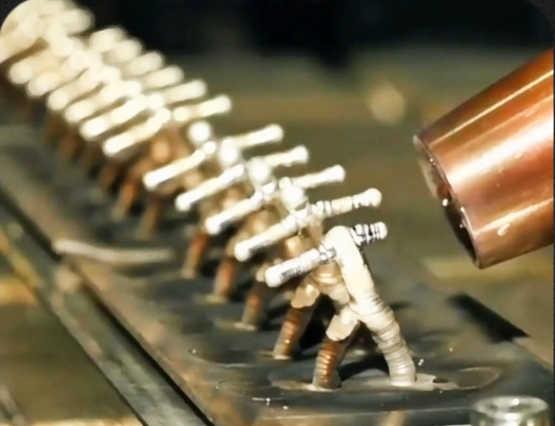
Innovatively, Zhang Haiou and team [1]devised the “Intelligent Micro-Casting, Forging, and Milling Hybrid Manufacturing” technique (as depicted in Figure 2). This approach blends electric arc micro-casting for additive shaping with continuous micro-forging, milling for subtractive shaping, and synchronously employing continuous micro-forging in the semi-solidified micro-zone of the deposition layer to refine grain structures. Subsequently, CNC milling is utilized to remove challenging and defective parts. This process offers advantages like a shortened workflow, reduced energy consumption, integrated and compact equipment, and superior mechanical properties compared to traditional forgings, marking it as a novel eco-friendly manufacturing method.
Furthermore, Akula et al. [2] and Karunakaran et al.[3] have developed a hybrid machine tool incorporating CNC milling and Gas Metal Arc Welding (GMAW). During hybrid processing, material deposition alternates with milling, and once near-net shape is achieved, precision milling is conducted to complete the component. Testing revealed that while components manufactured using this method may not match traditional manufacturing methods in mechanical performance, their geometric precision can reach ±0.030 mm after CNC milling. The team also analyzed the influence of deposition parameters in hybrid additive-subtractive manufacturing, revealing the variance in material microstructure with changing deposition parameters, along with deformations occurring in structural components during the additive process due to uneven temperature fluctuations.

Karunakaran et al. [3] and Sreenathbabu et al. [4] introduced a hybrid manufacturing technique utilizing electric arc melting and milling, termed Arc Hybrid-Layered Manufacturing (ArcHLM). After each deposition layer is completed, CNC end milling is employed to achieve a smooth surface, promoting good interlayer adhesion. To avoid collisions during the additive nozzle’s movement, pneumatic brakes are incorporated into the hybrid machine. Additionally, the welding power supply is placed within the machine’s protective panel to enhance safety. To address the challenging cooling aspect in hybrid additive-subtractive processing, a porous fixture with Z-shaped cooling tubes is utilized to enhance heat dissipation during both the deposition and machining processes (as shown in Figure 3). The ArcHLM process proves time and cost-efficient in manufacturing metal tools and molds, integrating additive manufacturing and precision machining into a unified platform. This technique represents one of the early endeavors in the development of hybrid additive-subtractive processing machines.
In a related approach, Song et al. [5] integrated two Gas Metal Arc Welding (GMAW) torches adjacent to the spindle of a 3-axis machine. They performed alternating deposition and milling operations, resulting in components with a density exceeding 90%. After milling, the surface roughness (Ra) of the components was measured at 2 μm, with tensile strength comparable to low-carbon steel wire. Song et al. [6] further utilized this setup to manufacture multi-material components by employing two additive nozzles to deposit different materials. For instance, they encapsulated a low-carbon steel core within a stainless steel shell, achieving a combination component with high strength and excellent toughness, evident through distinct interfaces observable under an electron microscope.
Currently, arc-based hybrid additive-subtractive manufacturing predominantly utilizes 3-axis or 5-axis machine tools or robots as the motion platform, retrofitting them with modified welding equipment for material deposition. To prevent hindrances during CNC machining caused by the welding torch, some devices incorporate telescopic functionality into the torch. However, this method typically focuses on milling the top surface of the deposition layer and lacks attempts at milling the interlayer contours. Consequently, components manufactured using this technique possess relatively simple structures without suspended or intricate internal features.
- Hybrid Manufacturing Utilizing Laser as an Energy Source
The laser-based 3D printing is a more popular additive manufacturing method in industry. There are some research and studies on hybrid manufacturing utilizing laser as an energy source.
Kerschbaumer and Ernst [7] reported a hybrid system integrating laser cladding with a CNC machine. They integrated an Nd:YAG laser cladding head and powder delivery system into a commercial Röders 5-axis CNC machine. The 5-axis capability allows material deposition in multiple build directions, reducing issues related to material flow on inclined surfaces and considerably lowering support structure demands. The flexibility of the 5-axis machine also expands the tool’s access area during subtractive processes. However, this study found that due to the stringent requirements of powder material for the cladding environment, cutting fluids cannot be utilized during the process, significantly reducing the forming efficiency.
Jeng and Lin [8] employed a combination of selective laser cladding (SLC) and milling to fabricate metal rapid prototypes and molds. This approach involves direct laser-induced melting of metal powder sprayed from a nozzle to form a cladding layer. Subsequently, the top and side surfaces of the cladding layer are milled to achieve the desired precision. The completed molds were used for injection molding, demonstrating satisfactory geometric precision and density.
Utilizing Selective Laser Melting (SLM) to construct delicate overhanging structures in metal additive components presents a major challenge. These fine external structures are fragile and struggle to withstand the impact of subsequent powder layers. Traditional subtractive methods find it difficult to handle such features. To address this, Yasa et al. [9] introduced a unique hybrid manufacturing approach. They operated an Nd:YAG laser in two different modes on the SLM equipment to execute two distinct manufacturing processes. The first is the additive SLM process, where the laser operates in continuous mode. The second is Selective Laser Erosion (SLE), utilizing a pulsed laser after the SLM process to remove excess material from the workpiece. This research showed that combining SLM and SLE effectively improved the surface roughness and porosity of SLM parts. Additionally, integrating SLE enhanced the precision processing capability of SLM, allowing for the creation of intricate internal and external structures in the 50–100 μm size range. This study demonstrated that a hybrid processing approach could be achieved by merely reconfiguring the machine settings through parameter changes, highlighting that hybrid processing involves more than just assembling different hardware modules from various processes. A single machine, without modification, can potentially achieve hybrid processing through different machining methods.
Du et al. [10] and Bai et al. [11] combined Selective Laser Melting (SLM) with precision milling techniques, utilizing 18Ni maraging steel as the raw material for additive-subtractive hybrid manufacturing. The resulting parts exhibited superior performance, accuracy, and surface quality compared to parts manufactured using forging and additive manufacturing methods. They found that parts post-milled after additive manufacturing had smaller residual stresses introduced during the solidification process compared to additive-only parts. Li et al. [12] designed and constructed China’s first self-made university-based 5-axis laser cladding and milling additive-subtractive hybrid equipment. They successfully manufactured, processed, and repaired metal parts. The Powder Metallurgy Research Institute of Central South University employed the first domestic hybrid additive-subtractive processing center, DMG MORI LASERTEC 65 3D, to complete additive-subtractive manufacturing of stainless steel powder. This process combined laser deposition and 5-axis CNC milling, resulting in parts with mechanical properties comparable to forgings and achieving precision manufacturing of complex-shaped parts such as anisotropic turbocharger housings.
Grinding is also an integral part of hybrid processing. Löber et al. [13] reduced the surface roughness of 316L stainless steel additive parts from 15 μm to 0.34 μm using grinding. Rossi et al. [14] reported that post-grinding, the surface roughness of Ni-Fe-Cu parts decreased from 12 μm to 4 μm on horizontal surfaces and from 15 μm to 13 μm on vertical surfaces. The complex geometries of additive parts pose a challenge to traditional grinding. To address this, Beauchamp et al. [15] used shape-adaptive grinding for post-processing Ti6Al4V metal additive manufacturing parts. This technique employed three different diamond abrasive grains to grind the part surface, achieving a surface roughness of 10 nm.
Sitthi-Amorn et al. [16] developed a hybrid additive-subtractive manufacturing device named MULTIFAB. The core of this system is a robotic arm for material deposition, which can be integrated into another 5-axis machine. The system is suitable for energy-direct deposition (DED) processes using energy sources such as arc, laser, and plasma. The primary function is workpiece scanning modeling, enabling high-value metal part repairs through reverse engineering of the workpiece’s geometric shape.
Currently, laser-based hybrid manufacturing dominates the hybrid manufacturing landscape. Due to the highly controllable nature of laser energy sources, when combined with subtractive techniques, they can manufacture components with the highest precision and the most complex shapes. Consequently, these methods have been applied by some companies for the precision repair of parts. Numerous well-known machine tool manufacturers, such as Hermle and Mazak, have also successively developed a large number of laser-based additive-subtractive hybrid machines. However, laser-based hybrid manufacturing encounters certain challenges. While theoretically, lasers can be used for additive manufacturing of most metal materials, high-reflectivity materials have lower energy absorption efficiency. Additionally, the use of cutting fluids during laser cladding is challenging, and the lack of effective heat dissipation methods can affect processing efficiency.
Hybrid Manufacturing Based on Alternative Energy Sources
Xiong et al. [17] proposed a Hybrid Plasma Deposition and Milling (HPDM) process (as shown in Figure 4). This process utilizes a plasma arc to melt the powder to form a deposited layer. Subsequently, milling is performed on the top surface to achieve a smooth surface with a certain thickness, facilitating further deposition. T-slot milling is used to shape the inner and outer surface contours, removing surface residues and obtaining a fine surface quality for nearly net-shaped metal parts. HPDM is challenging to manufacture typical turbine blades on a conventional three-axis machine due to the intricacy of the geometry. The process has been used to manufacture twisted blades. Penetrating inspection revealed no cracks, pores, or defects on the surface. The HPDM process involves high-temperature dry milling without the use of lubricants and coolants, leading to a rapid increase in friction between the tool, workpiece, and waste material. The increased cutting force elevates cutting energy rapidly, raising the temperature in the working area, and significantly reducing tool life. Research indicates that cooling with air can significantly reduce tool wear and extend tool life.
Zhu et al. [18] integrated Fused Deposition Modeling (FDM), CNC milling, and inspection devices to manufacture components using thermoplastic materials on a single platform. During the forming process, the part experienced deformation and reduced quality. This was due to the components being decomposed into individual sub-components built one by one and then assembled during the manufacturing process. Residual stresses were induced due to the temperature difference between the newly deposited sub-component (205°C) and the previously manufactured sub-component (20°C), causing part deformation. The research group plans to establish a Finite Element Analysis (FEA) model to simulate 3D transient heat conduction and convection based on different deposition patterns and tool paths, predicting the degree of deformation and part tolerances. This research is critical for optimizing this emerging hybrid manufacturing process.
Fabrisonic’s Ultrasonic Additive Manufacturing (UAM) technology is a unique metal additive manufacturing technique [19]. In the UAM process, ultrasonic waves are used to melt layers of metal pulled from ordinary metal sheets, achieving additive manufacturing of metal components. This method can achieve true metallurgical bonding and can use various metal materials such as aluminum, copper, stainless steel, and titanium, among others. Fabrisonic’s method can simultaneously ‘print’ multiple metal materials. The process can use rolled aluminum or copper metal foil to manufacture metal components with highly complex internal channels. In the UAM manufacturing process, ultrasonic waves with a frequency of up to 20 kHz are applied to the metal sheet. The oscillating energy of the ultrasonic waves causes friction between the two surfaces to be welded, creating molecular fusion between layers. Then, using the same principle, metal sheets are continuously welded layer by layer. Subsequently, fine 3D shaping is achieved through mechanical machining, resulting in a solid metal object.
Cold spraying is a unique additive manufacturing process that utilizes high-pressure gas to supersonically jet ultrafine powder materials onto the surface of a substrate or target workpiece. The particles bond and diffuse with the part’s surface to repair or shape it. This process doesn’t require the metal to be melted, thus avoiding the introduction of thermal stress and metastable phases. However, composite material coatings produced by cold spraying lose much of their ductility due to excessive dispersion strengthening. This is attributed to the uneven distribution of reinforcing particles and a noticeable interface between the coating and the substrate. Peat et al. [20] sought to address this issue by studying the combination of cold spraying with friction stir processing. Their research showed that the shear forces generated by friction stir processing significantly refined the reinforcing particles, resulting in a more uniform distribution. Additionally, grain boundary segregates were dispersed after the treatment. The treated coating exhibited a 120% increase in hardness and a substantial improvement in corrosion resistance. Courbon et al. [21] subjected cold-sprayed titanium alloy coatings to ball milling, and the results indicated a transition from tensile to compressive stress on the coating surface.
In the aforementioned hybrid manufacturing technologies, plasma arc shares similarities with electric arc in terms of advantages and drawbacks. Here, we won’t delve further into these similarities. Melting deposition forming is a prominent technique in plastic additive manufacturing, but it is prone to wire breakage when using materials with lower toughness. Ultrasonic additive manufacturing is limited by power and can only be used for thin foils. It is not ideal for thick plates or high-strength materials. Cold spraying technology has gained extensive research and application in recent years due to its numerous advantages. This technique can achieve spraying of various materials such as ceramics, polymers, and metals. When combined with subtractive techniques, cold spraying holds immense promise as a hybrid manufacturing technology. It is poised to play a crucial role in coating preparation for defense and aerospace applications.
Potential Applications in Various Industries
Hybrid additive-subtractive manufacturing is not yet widely prevalent in the industry. However, its potential applications are extensive.
- Repair of High-Value Components:
In cases where high-value components are damaged, hybrid manufacturing can be used to repair the damaged areas through additive manufacturing and then ensure dimensional accuracy through subtractive manufacturing.
- Manufacture of Complex High-Value Components:
Industries like aerospace often require intricate components with complex shapes, high surface finish, and precision. Examples include certain airways, ducts, and bifurcation joints. Similarly, certain components in the medical device industry can utilize additive manufacturing to add internal supports for weight reduction, while subtractive manufacturing can maintain assembly precision and surface roughness.
- Prototype Molds:
In the realm of low-volume plastic part mold manufacturing, although additive manufacturing theoretically allows for the direct production of mold cores and cavities, the precision of 3D printed mold cores and cavities may not suffice, posing challenges during mold assembly. Hybrid additive-subtractive manufacturing can significantly reduce the production time of prototype molds and address this issue.
- Prototyping of Complex-Shaped Parts Requiring Precision:
In the automotive industry and other sectors, there are requirements for components that are both intricate in shape and demand assembly precision, making them difficult to machine using CNC. Additionally, there is a need for rapid prototyping. In such cases, hybrid additive-subtractive manufacturing becomes an excellent choice.
This approach combines the benefits of both additive and subtractive manufacturing, promising an effective solution for creating high-value components and prototypes with precision, efficiency, and reduced lead times.
Current Market Landscape: Equipment for Hybrid Additive-Subtractive Manufacturing
Several prominent manufacturers have made strides in developing and commercializing these hybrid machines. Notable companies in this domain include:
- DMG Mori: A global leader in manufacturing machinery, DMG Mori offers hybrid machines that seamlessly combine CNC milling with laser metal deposition (LMD) for additive manufacturing.
- Mazak: Renowned for its CNC machinery, Mazak has innovated hybrid multitasking machines, integrating traditional CNC machining with laser metal deposition for a comprehensive manufacturing approach.
- Optomec: Specializing in additive manufacturing solutions, Optomec provides hybrid machines that integrate 3D printing and CNC capabilities, particularly geared towards repairing and producing complex parts.
- Hybrid Manufacturing Technologies: This company specializes in retrofitting existing CNC machines, enhancing them with hybrid capabilities that seamlessly integrate 3D printing functionality.
These hybrid CNC machines offer manufacturers unparalleled flexibility, allowing a smooth transition between subtractive and additive processes within a single machine. This integration enables efficient production, shortened lead times, and expanded design possibilities, catering to diverse applications across various industries.
Key Technological Challenges and Solutions
Hybrid additive-subtractive manufacturing presents a promising paradigm but is not without its technological challenges. Overcoming these hurdles is critical to realizing the full potential of this manufacturing approach.
- Development of Hybrid Equipment:
Challenge: Integrating both processes within a single machine necessitates addressing issues such as manufacturability, part support, material utilization, machine flexibility, component miniaturization, and part balance during the forming process.
Solution: Consideration of the interplay of numerous error factors, including reverse positioning errors, work plane errors, relative errors between axes, and errors in linked interpolation movements, to resolve contradictions between print quality and forming efficiency.
- Development of Online Inspection Systems:
Challenge: To precisely control changes in part accuracy and temperature gradients resulting from the composite process, real-time monitoring of material composition and morphology coefficients in the melt pool and deposition layers is necessary.
Solution: Implement sensors to detect expansion or shrinkage during part printing, enabling real-time adjustments in output power, powder feeding rate, overlap rate, milling depth, milling speed, and feed rate to maintain part continuity and integrity.
- Software Development for Intelligent Planning:
Challenge: For intricate components with internal holes, cavities, or channels, the complex positional relationships between layers pose challenges of interference and collisions.
Solution: Develop feature-based slicing and process-related software to intelligently plan the most optimal forming paths. Consideration of comprehensive factors like the compatibility of support structures with the hybrid process, the impact of machine rigidity on parts, the appropriate nozzle movement trajectory, real-time temperature monitoring during subtractive processing, and effective tool path planning.
- Integration of Support Structures:
Challenge: Determining factors for support structures, including their vertical angle compatibility with the hybrid process, the impact of machine rigidity on parts, structural strength of the printed design, suitable nozzle movement trajectory, real-time temperature monitoring during subtractive processing, and appropriate machine arm flexibility.
Solution: Tailoring support structures to achieve optimum balance between efficiency and surface quality and considering multi-factor aspects for comprehensive support planning.
Metal hybrid additive-subtractive manufacturing, built on the foundation of additive manufacturing and subsequent part subtraction, is an efficient, flexible, cost-effective, and high-precision green manufacturing technology. Overcoming the above-mentioned technological challenges is expected to unlock the immense potential of this technology, particularly in high-end sectors such as aerospace, energy, satellites, and smart manufacturing, meeting critical national strategic needs.
AI applications in the CNC machining industry
Future industrial potential
Introduction
Artificial intelligence (AI) and machine learning (ML) are significant advancements in computer science, benefiting various tech services, products, and industries. Machine learning, a subset of AI and computer science, uses data and algorithms to help machines learn and improve accuracy. It’s used to predict cutting forces and tool wear in CNC machines, prolonging tool life. Advanced machine learning optimizes machining parameters, boosting manufacturing efficiency and predicting surface quality for better-machined parts. Machine learning is also used to predict and reduce power consumption during CNC machining. The article reviews these applications and suggests future research, aiming to advance the use of AI and ML in CNC machines.
CNC machining is a crucial method for making parts. It’s like the engine of modern manufacturing. Industries like automotive, medical, aerospace, and more use CNC machining for creating different parts. CNC machinery has revolutionized manufacturing, helping businesses achieve their goals. However, as manufacturing changes and new technology comes in, we need to think about the future of CNC machining. Machine learning (ML) helps computers learn from data and predict things without people. The combination of the two may be the future CNC machining trend.
General concepts from past studies
AI and machine learning impact CNC machining’s future for manufacturing [22]. They improve machine performance using real-time data and analytics [23, 24]. Advanced sensors enable better tool condition monitoring [25]. AI enhances productivity and efficiency in CNC operations [26]. Machines analyze data and provide real-time insights [27]. AI improves decision-making, reducing downtime [28].
CNC operations need optimizing for cost savings and increased profits [29]. AI predicts servicing needs and improves tool life using production data [30, 31]. Deep learning enhances machining monitoring and defect detection [32, 33]. Machine and deep learning optimize operation management and sustainable manufacturing [34, 35]. They also improve smart manufacturing systems and offer future directions [36, 37]
Soori et al. improved CNC machining digitally using virtual methods [38-41]. They also reviewed friction stir welding for efficient component manufacturing [42]. Their work reduced errors and improved milling processes for various components [43-49]. They also examined and enhanced process planning for better productivity [50, 51]. Dastres et al. researched RFID-based systems to enhance energy efficiency and data quality in manufacturing [52].
Dastres and Soori discuss advancements in web-based decision support systems [53]. They also review the use of artificial neural networks across various fields for performance enhancement [54]. Additionally, they highlight leveraging technology to mitigate natural disaster impacts [55]. For improved security in networks and data webs, they introduce the secure socket layer [56]. The duo further explores recent developments in web-based decision support systems and network security measures [57, 58]. They also delve into advancing image processing systems for varied applications [59].
The upcoming section will explore various applications of ML and AI in CNC machining operations.
The AI model helps reduce machine downtime
AI Optimization of CNC machining parameters
ML Cutting tool wear prediction
AI-based Cutting force model
ML helps with CNC machine maintenance
AI helps monitor machining operations
AI-based Surface quality prediction
AI-based Energy prediction systems
The AI model helps reduce machine downtime.
Predicting and minimizing machine downtime is crucial for the industry, allowing for proactive maintenance scheduling, smoother production planning, and fewer production delays.
The main concept involves gathering a comprehensive set of data (e.g., machine operating time, current usage, vibration, processed material) and analyzing and identifying relevant data or combinations. The AI model then establishes predictions for when and how likely the machine is going to experience a malfunction.
A recent approach to predicting machine downtime follows this workflow [60]:
- Sensor Embedded Physical System: The initial step involves collecting data using sensors that cover potential causes of machine downtime. The choice of sensors significantly impacts the relevance of collected data to machine downtime.
- Data Collection & Processing: This stage involves collecting, saving, and processing data (removing anomalies, for instance). Data comprises sensor readings and other pertinent operational information like the last maintenance time.
- Feature Extraction: Identifying relevant data is crucial and can be achieved through statistical analysis or AI models.
- Model Development: Knowing the relevant features, machine learning (ML) is used to establish a model using all available data and meaningful features. The model should predict the likelihood of machine downtime based on new input data.
- RUL Prediction (Remaining Useful Life): Remaining Useful Life (RUL) estimates how many more years a component in a production line is expected to function correctly before requiring replacement. The developed model from Step 4 helps make an accurate prediction.
- Decision Making: In this final step, the production manager uses various factors like maintenance cost, potential production loss due to downtime, and the likelihood of machine downtime to decide when or if to perform preventive maintenance.”
This prediction algorithm is based on data-driven. With the development of the Internet of Things, data collection will become more convenient, and huge amounts of data collection and analysis will become possible. It is foreseeable that data-driven prediction algorithms will be widely used in the future.
AI Optimization of CNC machining parameters
In the CNC machining industry, how to optimize processing parameters to obtain the highest profits is an eternal topic. There are 2 approaches to solving the topic.
Multi-objective optimization
The basic idea here is that the object of optimization machining parameters is not one but several. The process should be fast, with less tool wear, no risk of machine damage, less tool cost, etc. From a machine shop management point of view, the purpose has to include maximum total production volume, maximum using full capacity, etc. In the computer science world, this is a typical Multi-objective optimization problem. To optimize machining conditions and performance, a generalized technique for multi-response machining process optimization employing machine learning and genetic algorithms is developed [61].
One target optimization
A simpler approach exists for optimization. Factors like machining speed, tool wear, risk of machine damage, and more can all be assessed in terms of loss or profit using engineering and operational knowledge. Subsequently, the primary optimization goal is straightforward: maximizing profit.
Various studies have adopted this approach and employed different machine-learning algorithms. Examples include the Hybrid Differential Evolution Algorithm, Genetic Algorithm, Particle Swarm Optimization, Shuffled Frog-Leaping Algorithm, and Nelder-Mead Simplex Method.
The table below illustrates the results of a case study, demonstrating varying profits achieved by applying different algorithms to optimize parameters for the machining operation.
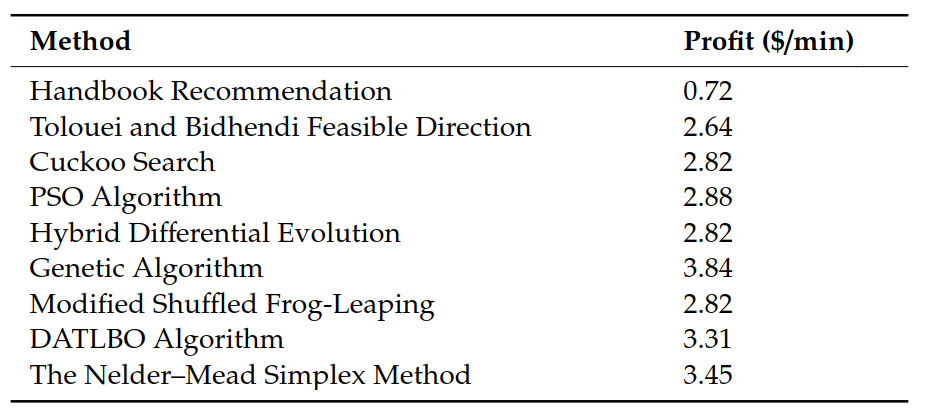
ML Cutting tool wear prediction
Machine learning is a powerful tool for predicting tool wear in complex processes. Artificial Neural Networks (ANNs) are preferred for this due to tool wear’s non-linear nature. Modern sensors and computational intelligence help monitor tool health, preventing issues. Monitoring tool health has become vital for extending cutting tool lifespan during machining [62]. Monitoring strategies fall into ‘Offline/Direct’ and ‘Online/Indirect’ methods. Direct methods suit complex failure (hard faults) analysis but are unsuitable for machine learning [63, 64]. Adaptive Neuro-Fuzzy Inference Systems enhance ‘Online/Indirect’ tool wear prediction during turning, as shown in Figure 3 [65].

In various studies, researchers have used advanced technology like deep learning to estimate how cutting tools wear out during milling [66]. For instance, in face milling, a smart system called a deep neural network detects tool wear while chips are being made [67]. Another study focused on drilling operations and used a complex approach called adaptive neuro fuzzy-genetic algorithm to make sure the drills last as long as possible [68]. Additionally, some studies developed advanced neural network systems to accurately predict tool wear based on the energy used during CNC machining [69]. Simple machine learning and data-driven methods were also used to monitor tool wear during machining [70]. These technologies help enhance product quality and save costs during metal-cutting operations [71]. Cloud-based parallel machine learning was utilized to estimate tool wear and extend the tool’s life during machining operations [72]. Researchers compared different machine learning methods to predict tool wear in smart factories [73]. They also used sound waves and other smart methods to assess tool wear during milling under different conditions [74]. Machine learning helped predict the cutting tool’s condition in a vertical machining center [75]. Calibration-based monitoring helped predict how tools wear out during the milling process [76]. Acoustic emission signals were used in a novel machine learning-based method to estimate tool wear during milling operations [77]. Machine learning was also used to estimate wear considering specific conditions like wear on face mill teeth, improving accuracy [78]. Lastly, artificial neural networks were developed to evaluate tool wear on a modified CNC milling machine [79]. All these advancements help analyze and improve cutting tool life during the milling process for different materials and machining conditions using machine learning and AI.
The procedure of a methodology using ML in the tool wear prediction is shown in Fig. 4 .

AI-based Cutting force model
The force applied during cutting is crucial for how well milling works and the quality it produces. Modern computer systems, known as Machine Learning (ML) systems, can accurately predict this cutting force [80]. In milling, a combined approach using ML has been developed to analyze and understand forces during cutting [81]. Various ML methods, such as support vector regression and random forest, are used to estimate cutting forces accurately in milling [82]. In high-speed turning, ML helps predict cutting forces, surface roughness, and how long the tools will last [83]. Another hybrid technique uses ML and traditional methods to predict cutting forces while considering tool wear [84]. For CNC turning, a technique using wavelet packet analysis helps assess surface quality during cutting [85]. A smart tool holder, calibrated using ML, helps measure cutting forces accurately in precise turning operations [86]. Real-time measurements and a machine learning approach (CNN) help categorize tool wear during machining [87]. Machine learning with signal analysis is used to determine tool damage during machining [88]. These advances using ML and AI improve the accuracy and adaptability of models predicting cutting forces during CNC machining.
ML helps with CNC machine maintenance
Maintaining CNC machines takes time and money. One of the toughest challenges is predicting when to calibrate, modify components, and service these machines [89]. Machine learning and artificial intelligence are closely linked to this maintenance, aiming to predict issues and reduce downtime, boosting productivity [90]. Machine learning can accurately foresee when maintenance is needed, minimizing downtime and costs [91]. By using real-time data and automatic warnings, machine maintenance can be managed effectively to maintain a smooth workflow and production process [92]. Artificial intelligence can establish cause-and-effect connections, enhancing decision-making for CNC machine production [93]. A hybrid approach using digital twin technology is presented to accurately predict maintenance needs during CNC machine-based part production [94]. The hybrid approach method is illustrated in Figure 6.
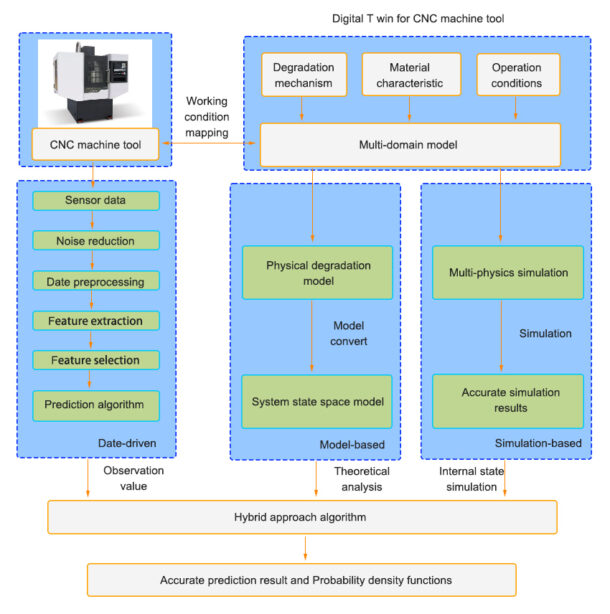
Advanced machine learning systems are developed to evaluate maintenance operations, including monitoring tool wear in CNC machine tools [91]. Sophisticated machine learning systems are utilized to monitor and assess CNC machine tool and cutting process conditions [95]. A tool health monitoring system is created using machine learning techniques during end milling to extend cutting tool life and enhance production efficiency [96]. Applying ML and AI to CNC machine tools’ working time during part production leads to advanced CNC machine tool maintenance procedures.
AI helps monitor machining operations
Recently, machine learning is being used to monitor the health of CNC machine tools, improving part production efficiency through machining operations [97]. Monitoring the condition of CNC machine tools is crucial for safe and reliable machining operations [98]. A smart monitoring system for CNC cutting tools is designed using a cyber-physical manufacturing and engineering structure [99]. This system combines physical and virtual modeling of the milling process, as shown in Figure 7 [99].
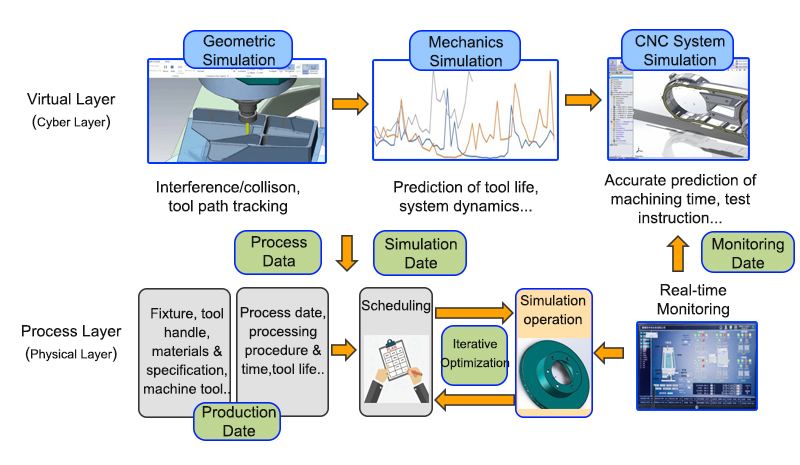
An advanced decision-making tool is introduced to monitor CNC machine tool performance during part production. Using a sophisticated machine learning system, the impact of process parameters (e.g., cutter speed, feed rate, and depth of cut) on outputs in turn-milling operations is studied [100]. Enhancing accuracy during movement, six rotating sensors on joints of three legs are used in the Stewart structure [101]. A monitoring system is implemented using adaptive neuro-fuzzy integration of multi-sensor signals to detect and prevent cutting tool errors during CNC machining operations [102]. For improved accuracy in CNC machining, a method using virtual reality and digitized twin systems for monitoring machining processes is developed [103].
Online monitoring systems are utilized for planning and optimizing machining parameters, focusing on material removal rate during AISI P20 milling operations to minimize manufacturing time [104]. Productivity in machining tough materials is enhanced using machine learning approaches like decision trees and artificial neural networks for chatter predictions in titanium alloy high-speed milling [105]. Predicting tool wear during machining operations is developed using machine learning techniques and force analysis based on spindle speed and feed rate parameters [106]. To optimize CNC machining parameters, a technique using response surface and genetic algorithm is employed [107]. Enhancing machine tool monitoring systems’ capabilities and accuracy, applications of artificial neural networks are introduced [108]. In summary, using machine learning and AI, data analysis during machining operations is greatly improved, enhancing decision-making in computer-aided process planning systems.
AI-based Surface quality prediction
Surface roughness is a vital measure of product quality. Advanced machining aims for precise shapes and smooth surfaces while reducing costs. Some traditional methods fall short, requiring additional surface finishing for quality. Surface roughness is a key factor in product quality, impacting part lifespan [109]. AI, especially Neural Networks, helps predict and analyze surface finish of machined parts [110]. Machine learning algorithms accurately predict surface quality in turning operations [111]. Techniques like linear regression, random forest, and decision trees predict surface quality of machined parts [112]. Data-driven approaches predict machining accuracy and surface quality for CNC machines [113]. Deep learning neural networks using vibration signals analyze and predict surface roughness during machining [114]. The methodology for applying AI in predicting surface roughness is shown in Figure 8 [114].
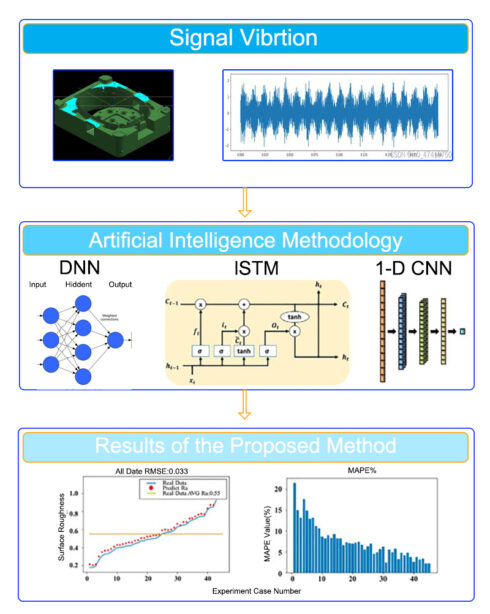
To improve surface quality in machining, a system predicts roughness based on tool wear [115]. Neural network models enhance roughness prediction in aluminum alloy machining [116]. Machine learning analyzes cutting forces in milling for better accuracy [117]. Systems measure surface roughness for precise production and quality [118]. Figure 9 shows an advanced surface measurement system [118].
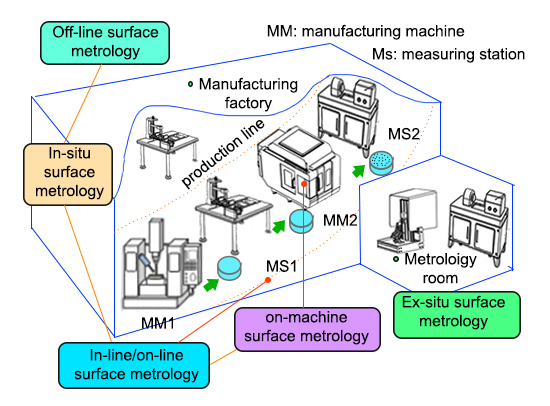
Machine learning algorithms on a milling machine monitor and improve surface quality in real-time [119]. Hybrid machine learning predicts cutting tool conditions using multimodal data [120]. A deep learning system detects tool wear, extending tool life [121]. Using advanced methods, a nested-ANN model predicts surface roughness in machined parts by considering cutting forces and tool oscillations [122]. Applying ML and AI enhances surface prediction, improving productivity in CNC machining operations [122].
AI-based Energy prediction systems
In various studies, researchers emphasize reducing energy waste in industrial production by focusing on building energy prediction and management systems [123, 124]. Machine learning techniques are now used to predict energy consumption during machining operations, significantly enhancing the accuracy, durability, and precision of traditional time series forecasting methods [125]. An advanced energy consumption model is proposed using a method that embeds deep learning and semi-supervised learning [126]. Strategies based on AI and machine learning in energy management systems improve the accuracy of energy consumption prediction [127]. Quick and accurate forecasts of CNC machining energy usage are crucial for efficient energy management and ensuring long-term sustainability in the manufacturing industry [128]. Advanced deep learning-based methods are developed to enhance energy prediction during machining processes [129]. Figure 10 illustrates the overall framework of this approach [129].
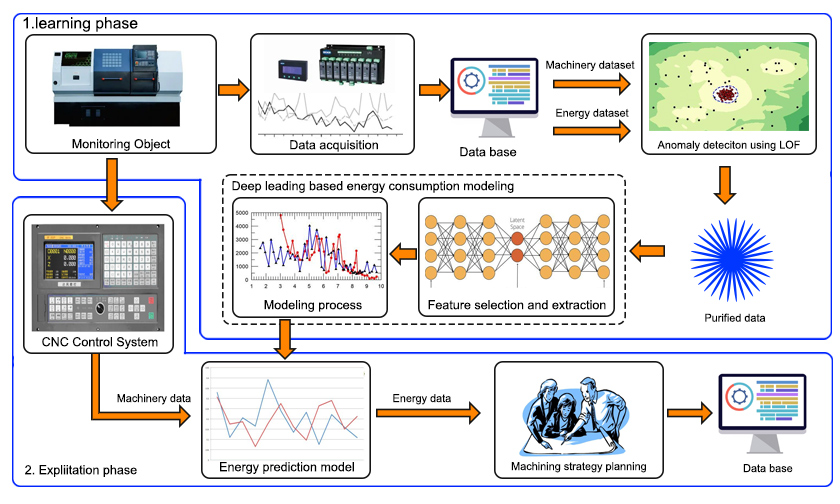
A hybrid method using machine learning and process mechanics is created to estimate cutting power during CNC machining [130]. Advanced machine learning, like data-driven simulation, predicts energy consumption in five-axis process planning [131]. Techniques using machine learning, like random forest and time series forecasting, are developed to predict energy usage in the machine tool spindle during machining [132]. Integrated methods combining process planning and cutting parameter optimization aim to minimize power consumption in CNC machining [133]. Optimal CNC turning process variables are determined to save energy and ensure high-quality machining [134]. Applying ML and AI in predicting energy consumption during chip formation improves productivity in part production using CNC machining [134].
Conclusion
AI is being used in CNC machining to make things work better and save money, just like in other industries. Many studies have looked into how AI can be used in CNC machining, and they’ve found some good ideas. But, most of these studies are still in the thinking and trying things out stage, and not many have been used in real factories yet. Just like with other new things, AI needs more testing and figuring out to really be useful in CNC machining.
Hybrid additive-subtractive manufacturing offers a promising approach to overcoming various challenges in the manufacturing industry. By seamlessly integrating additive and subtractive processes, this technology opens new doors for fabricating complex components and optimizing production. The potential applications in sectors like aerospace, medical, molds, and beyond underscore the vast possibilities that lie ahead. Embracing the advancements and addressing the technological hurdles in this field will undoubtedly contribute to a more efficient, flexible, and precise manufacturing landscape.
In summary, CNC manufacturing is changing fast, with cool tech like AI, HASM and automation making things better. These changes mean products are being made more accurately and quickly.
References:
- [1] Zhang H O, Huang C, Li R S, et al. A super short-process green manufacturing method and energy consumption analysis of micro casting forging and milling for high performance parts. China Mech Eng, 2018, 29(21): 2553
- [2] Akula S, Karunakaran K P. Hybrid adaptive layer manufacturing: an intelligent art of direct metal rapid tooling process. Robot Comput-Integr Manuf, 2006, 22(2): 113
- [3] Karunakaran K P, Suryakumar S, Pushpa V, et al. Retrofitment of a CNC machine for hybrid layered manufacturing. Int J Adv Manuf Technol, 2009, 45(7): 690
- [4]Sreenathbabu A, Karunakaran K P, Amarnath C. Statistical process design for hybrid adaptive layer manufacturing. Rapid Prototyping J, 2005, 11(4): 235
- [5] Song Y A, Park S, Choi D, et al. 3D welding and milling: Part I-a direct approach for freeform fabrication of metallic prototypes. Int J Mach Tools Manuf, 2005, 45(9): 1057
- [6] Song Y A, Park S, Chae S W. 3D welding and milling: Part II – Optimization of the 3D welding process using an experimental design approach. Int J Mach Tools Manuf, 2005, 45(9): 1063
- [7] Kerschbaumer M, Ernst G. Hybrid manufacturing process for rapid high performance tooling combining high speed milling and laser cladding // Proceedings of the 23rd International Congress on Applications of Lasers & Electro-Optics. Orlando,2004: 1710
- [8] Jeng J Y, Lin M C. Mold fabrication and modification using hybrid processes of selective laser cladding and milling. J Mater Process Technol, 2001, 110(1): 98
- [9] Yasa E, Kruth J P, Deckers J. Manufacturing by combining selective laser melting and selective laser erosion/laser re-melting. CIRP Annals, 2011, 60(1): 263
- [10] Du W, Bai Q, Zhang B. A novel method for additive/subtractive hybrid manufacturing of metallic parts. Procedia Manuf, 2016, 5: 1018
- [11] Bai Q, Dong Z G, Yan Y, et al. Development of additive / subtractive hybrid manufacturing practical teaching platform for full-time master of engineering students. Lab Sci, 2018, 21(2):158
- [12] Li Q Y, Li D C. Zhang A F, et al. Development and challenges of laser cladding deposition and machining composite manufacturing technology // Proceedings of the 17th National Special Processing Conference (Abstract). Guangzhou, 2017: 194
- [13] Löber L, Flache C, Petters R, et al. Comparison of different post processing technologies for SLM generated 316L steel parts. Rapid Prototyping J, 2013, 19(3): 173
- [14] Rossi S, Deflorian F, Venturini F. Improvement of surface finishing and corrosion resistance of prototypes produced by direct metal laser sintering. J Mater Process Technol, 2004, 148(3): 301
- [15] Beaucamp A T, Namba Y, Charlton P, et al. Finishing of additively manufactured titanium alloy by shape adaptive grinding (SAG). Surf Topography: Metrology Prop, 2015, 3(2): 024001
- [16] Sitthi-Amorn P, Ramos J E, Wangy Y, et al. MultiFab: a machine vision assisted platform for multi-material 3D printing. ACM Trans Graph, 2015, 34(4): 129
- [17] Xiong X H, Zhang H O, Wang G L. Metal direct prototyping by using hybrid plasma deposition and milling. J Mater Process Technol, 2009, 209(1): 124
- [18] Zhu Z, Dhokia V G, Nassehi A, et al. A review of hybrid manufacturing processes – State of the art and future perspectives. Int J Comput Integr Manuf, 2013, 26(7): 596
- [19] Hehr A, Wenning J, Terrani K, et al. Five-axis ultrasonic additive manufacturing for nuclear component manufacture. JOM, 2017, 69(3): 485
- [20] Peat T, Galloway A, Toumpis A, et al. The erosion performance of cold spray deposited metal matrix composite coatings with subsequent friction stir processing. Appl Surf Sci, 2017, 396: 1635
- [21] Courbon C, Sova A, Valiorgue F, et al. Near surface transformations of stainless steel cold spray and laser cladding deposits after turning and ball-burnishing. Surf Coat Technol,2019, 371: 235
- [22] L.C. Moreira, W. Li, X. Lu, M.E. Fitzpatrick Supervision controller for real-time surface quality assurance in CNC machining using artificial intelligence Comput. Ind. Eng., 127 (2019), pp. 158-168
- [23] D.-.H. Kim, T.J. Kim, X. Wang, M. Kim, Y.-.J. Quan, J.W. Oh, S.-.H. Min, H. Kim, B. Bhandari, I. Yang Smart machining process using machine learning: a review and perspective on machining industry Int. J. Precis. Eng. Manuf.-Green Technol., 5 (2018), pp. 555-568
- [24] Z.M. Çınar, A. Abdussalam Nuhu, Q. Zeeshan, O. Korhan, M. Asmael, B. Safaei Machine learning in predictive maintenance towards sustainable smart manufacturing in industry 4.0 Sustainability, 12 (2020), p. 8211
- [25] P. Krishnakumar, K. Rameshkumar, K. Ramachandran Acoustic emission-based tool condition classification in a precision high-speed machining of titanium alloy: a machine learning approach Int. J. Comput. Intell. Appl., 17 (2018), Article 1850017
- [26] L. Li, C. Li, Y. Tang, Q. Yi Influence factors and operational strategies for energy efficiency improvement of CNC machining J. Clean. Prod., 161 (2017), pp. 220-238
- [27] S. Nallusamy Enhancement of productivity and efficiency of CNC machines in a small scale industry using total productive maintenance Int. J. Eng. Res. Africa, Trans. Tech. Publ. (2016), pp. 119-126
- [28] Y. Yang, T. Hu, Y. Ye, W. Gao, C. Zhang A knowledge generation mechanism of machining process planning using cloud technology J. Ambient Intell. Humaniz. Comput., 10 (2019), pp. 1081-1092
- [29] Y. Xiao, Z. Jiang, Q. Gu, W. Yan, R. Wang A novel approach to CNC machining center processing parameters optimization considering energy-saving and low-cost J. Manuf. Syst., 59 (2021), pp. 535-548
- [30] S. Wan, D. Li, J. Gao, J. Li A knowledge based machine tool maintenance planning system using case-based reasoning techniques Robot Comput. Integr. Manuf., 58 (2019), pp. 80-96
- [31] Z. Hussain, H. Jan Establishing simulation model for optimizing efficiency of CNC machine using reliability-centered maintenance approach Int. J. Model., Simul., Sci. Comput., 10 (2019), Article 1950034
- [32] V. Nasir, F. Sassani A review on deep learning in machining and tool monitoring: methods, opportunities, and challenges Int. J. Adv. Manuf. Technol., 115 (2021), pp. 2683-2709
- [33] J. Yang, S. Li, Z. Wang, H. Dong, J. Wang, S. Tang Using deep learning to detect defects in manufacturing: a comprehensive survey and current challenges Materials, 13 (2020), p. 5755
- [34] M. Bertolini, D. Mezzogori, M. Neroni, F. Zammori Machine Learning for industrial applications: a comprehensive literature review Expert Syst. Appl., 175 (2021), Article 114820
- [35] A. Jamwal, R. Agrawal, M. Sharma, A. Kumar, V. Kumar, J.A.A. Garza-ReyesMachine learning applications for sustainable manufacturing: a bibliometric-based review for future research J. Enterprise Inf. Manage., 35 (2021), pp. 566-596
- [36] J. Wang, Y. Ma, L. Zhang, R.X. Gao, D. Wu Deep learning for smart manufacturing: methods and applications J. Manuf. Syst., 48 (2018), pp. 144-156
- [37] A. Rajesh, M. Prabhuswamy, S. Krishnasamy Smart manufacturing through machine learning: a review, perspective, and future directions to the machining industry J. Eng. (2022), p. 2022
- [38] M. Soori, B. Arezoo, M. Habibi Accuracy analysis of tool deflection error modelling in prediction of milled surfaces by a virtual machining system Int. J. Comput. Appl. Technol., 55 (2017), pp. 308-321
- [39] M. Soori, B. Arezoo, M. Habibi Virtual machining considering dimensional, geometrical and tool deflection errors in three-axis CNC milling machines J. Manuf. Syst., 33 (2014), pp. 498-507
- [40] M. Soori, B. Arezoo, M. Habibi Dimensional and geometrical errors of three-axis CNC milling machines in a virtual machining system Comput. Aided Des., 45 (2013), pp. 1306-1313
- [41] M. Soori, B. Arezoo, M. Habibi Tool deflection error of three-axis computer numerical control milling machines, monitoring and minimizing by a virtual machining system J. Manuf. Sci. Eng., 138 (2016)
- [42] M. Soori, M. Asmael, D. Solyalı Recent development in friction stir welding process: a review SAE Int. J. Mater. Manuf. (2020), p. 18
- [43] M. Soori, M. Asmael Virtual minimization of residual stress and deflection error in five-axis milling of turbine blades Strojniski Vestnik/J. Mech. Eng., 67 (2021), pp. 235-244
- [44] M. Soori, M. Asmael Cutting temperatures in milling operations of difficult-to-cut materials J. New Technol. Mater., 11 (2021), pp. 47-56
- [45] M. Soori, M. Asmael, A. Khan, N. Farouk Minimization of surface roughness in 5-axis milling of turbine blades Mech. Based Des. Struct. Mach. (2021), pp. 1-18
- [46] M. Soori, M. Asmael Minimization of deflection error in five axis milling of impeller blades Facta Universitatis, Ser.: Mech. Eng. (2021)
- [47] M. Soori, B. Arezoo A review in machining-induced residual stress J. New Technol. Mater., 12 (2022), pp. 64-83
- [48] M. Soori, B. Arezoo Minimization of surface roughness and residual stress in grinding operations of inconel 718 J. Mater. Eng. Perform. (2022), pp. 1-10
- [49] M. Soori, B. Arezoo Cutting tool wear prediction in machining operations, a review J. New Technol. Mater., 12 (2022), pp. 15-26
- [50] M. Soori, M. Asmael Classification of research and applications of the computer aided process planning in manufacturing systems Independent J. Manage. Prod., 12 (2021), pp. 1250-1281
- [51] M. Soori, M. Asmael A review of the recent development in machining parameter optimization Jordan J. Mech. Ind. Eng., 16 (2022), pp. 205-223
- [52] R. Dastres, M. Soori, M. Asmael Radio Frequency Identification (RFID) based wireless manufacturing systems, a review Independent J. Manage. Prod., 13 (2022), pp. 258-290
- [53] R. Dastres, M. Soori Advances in web-based decision support systems Int. J. Eng. Future Technol., 19 (2021), pp. 1-15
- [54] R. Dastres, M. Soori Artificial neural network systems Int. J. Imaging Robot. (IJIR), 21 (2021), pp. 13-25
- [55] R. Dastres, M. Soori The role of Information and Communication Technology (ICT) in environmental protection Int. J. Tomogr. Simul., 35 (2021), pp. 24-37
- [56] R. Dastres, M. Soori Secure socket layer in the network and web security Int. J. Comput. Inf. Eng., 14 (2020), pp. 330-333
- [57] R. Dastres, M. Soori Advances in web-based decision support systems Int. J. Eng. Future Technol. (2021)
- [58] R. Dastres, M. Soori A review in recent development of network threats and security measures Int. J. Inf. Sci. Comput. Eng. (2021)
- [59] R. Dastres, M. Soori Advanced image processing systems Int. J. Imagining Robot., 21 (2021), pp. 27-44
- [60] Y. Wen, M.F. Rahman, H. Xu, T.-L.B. Tseng Recent advances and trends of predictive maintenance from data-driven machine prognostics perspective Measurement, 187 (2022), Article 110276
- [61] T. Ghosh, K. Martinsen Generalized approach for multi-response machining process optimization using machine learning and evolutionary algorithms Eng. Sci. Technol., Int. J., 23 (2020), pp. 650-663
- [62] S. Ravikumar, K. Ramachandran Tool wear monitoring of multipoint cutting tool using sound signal features signals with machine learning techniques Mater. Today: Proc., 5 (2018), pp. 25720-25729
- [63] V. Parwal, B. Rout Machine learning based approach for process supervision to predict tool wear during machining Procedia CIRP, 98 (2021), pp. 133-138
- [64] P.J. Bagga, M.A. Makhesana, A.D. Pala, K.C. Chauhan, K.M. Patel, A novel computer vision based machine learning approach for online tool wear monitoring in machining, (2021).
- [65] M. Rizal, J.A. Ghani, M.Z. Nuawi, C.H.C. Haron Online tool wear prediction system in the turning process using an adaptive neuro-fuzzy inference system Appl. Soft Comput., 13 (2013), pp. 1960-1968
- [66] X. Zhang, C. Han, M. Luo, D. Zhang Tool wear monitoring for complex part milling based on deep learning Appl. Sci., 10 (2020), p. 6916
- [67] X. Wu, Y. Liu, X. Zhou, A. Mou Automatic identification of tool wear based on convolutional neural network in face milling process Sensors, 19 (2019), p. 3817
- [68] L.H. Saw, L.W. Ho, M.C. Yew, F. Yusof, N.A. Pambudi, T.C. Ng, M.K. Yew Sensitivity analysis of drill wear and optimization using adaptive neuro fuzzy–genetic algorithm technique toward sustainable machining J. Clean. Prod., 172 (2018), pp. 3289-3298
- [69] A. Proteau, A. Tahan, M. Thomas Specific cutting energy: a physical measurement for representing tool wear Int. J. Adv. Manuf. Technol., 103 (2019), pp. 101-110
- [70] A. de Farias, S.L.R. de Almeida, S. Delijaicov, V. Seriacopi, E.C. Bordinassi Simple machine learning allied with data-driven methods for monitoring tool wear in machining processes Int. J. Adv. Manuf. Technol., 109 (2020), pp. 2491-2501
- [71] B. Lutz, D. Kisskalt, A. Mayr, D. Regulin, M. Pantano, J. Franke In-situ identification of material batches using machine learning for machining operations J. Intell. Manuf., 32 (2021), pp. 1485-1495
- [72] D. Wu, C. Jennings, J. Terpenny, S. Kumara, R.X. Gao Cloud-based parallel machine learning for tool wear prediction J. Manuf. Sci. Eng. (2018), p. 140
- [73] D. Wu, C. Jennings, J. Terpenny, R.X. Gao, S. Kumara A comparative study on machine learning algorithms for smart manufacturing: tool wear prediction using random forests J. Manuf. Sci. Eng. (2017), p. 139
- [74] A. Kothuru, S.P. Nooka, R. Liu Application of audible sound signals for tool wear monitoring using machine learning techniques in end milling Int. J. Adv. Manuf. Technol., 95 (2018), pp. 3797-3808
- [75] A.D. Patange, R. Jegadeeshwaran A machine learning approach for vibration-based multipoint tool insert health prediction on vertical machining centre (VMC) Measurement, 173 (2021), Article 108649
- [76] R. Liu, A. Kothuru, S. Zhang Calibration-based tool condition monitoring for repetitive machining operations J. Manuf. Syst., 54 (2020), pp. 285-293
- [77] J.L. Ferrando Chacón, T. Fernández de Barrena, A. García, M. Sáez de Buruaga, X. Badiola, J. Vicente A novel machine learning-based methodology for tool wear prediction using acoustic emission signals Sensors, 21 (2021), p. 5984
- [78] A. Bustillo, D.Y. Pimenov, M. Mia, W. Kapłonek Machine-learning for automatic prediction of flatness deviation considering the wear of the face mill teethJ. Intell. Manuf., 32 (2021), pp. 895-912
- [79] D.F. Hesser, B. Markert Tool wear monitoring of a retrofitted CNC milling machine using artificial neural networks Manuf. Lett., 19 (2019), pp. 1-4
- [80] V.F. Sousa, F.J. Silva, J.S. Fecheira, H.M. Lopes, R.P. Martinho, R.B. Casais, L.P. Ferreira Cutting forces assessment in CNC machining processes: a critical review Sensors, 20 (2020), p. 4536
- [81] S. Vaishnav, A. Agarwal, K. Desai Machine learning-based instantaneous cutting force model for end milling operation J. Intell. Manuf., 31 (2020), pp. 1353-1366
- [82] P. Charalampous Prediction of cutting forces in milling using machine learning algorithms and finite element analysis J. Mater. Eng. Perform., 30 (2021), pp. 2002-2013
- [83] Y. Zhang, X. Xu Machine learning cutting force, surface roughness, and tool life in high speed turning processes Manuf. Lett., 29 (2021), pp. 84-89
- [84] B. Peng, T. Bergs, D. Schraknepper, F. Klocke, B. Döbbeler A hybrid approach using machine learning to predict the cutting forces under consideration of the tool wear Procedia CIRP, 82 (2019), pp. 302-307
- [85] E.G. Plaza, P.N. López Analysis of cutting force signals by wavelet packet transform for surface roughness monitoring in CNC turning Mech. Syst. Signal Process., 98 (2018), pp. 634-651
- [86] L.-.W. Tseng, T.-.S. Hu, Y.-.C. Hu A smart tool holder calibrated by machine learning for measuring cutting force in fine turning and its application to the specific cutting force of low carbon steel S15C Machines, 9 (2021), p. 190
- [87] G. Terrazas, G. Martínez-Arellano, P. Benardos, S. Ratchev Online tool wear classification during dry machining using real time cutting force measurements and a CNN approachJ. Manuf. Mater. Process., 2 (2018), p. 72
- [88] G. Kucukyildiz, H.G. Demir A multistage cutting tool fault diagnosis algorithm for the involute form cutter using cutting force and vibration signals spectrum imaging and convolutional neural networks Arab. J. Sci. Eng., 46 (2021), pp. 11819-11833
- [89] A. Jimenez-Cortadi, I. Irigoien, F. Boto, B. Sierra, G. Rodriguez Predictive maintenance on the machining process and machine tool Appl. Sci., 10 (2019), p. 224
- [90] B. Luo, H. Wang, H. Liu, B. Li, F. Peng Early fault detection of machine tools based on deep learning and dynamic identification IEEE Trans. Ind. Electron., 66 (2018), pp. 509-518
- [91] E. Traini, G. Bruno, G. D’antonio, F. Lombardi Machine learning framework for predictive maintenance in milling IFAC-PapersOnLine, 52 (2019), pp. 177-182
- [92] J. Diaz-Rozo, C. Bielza, P. Larrañaga Machine learning-based CPS for clustering high throughput machining cycle conditions Procedia Manuf., 10 (2017), pp. 997-1008
- [93] S. Wan, D. Li, J. Gao, R. Roy, F. He A collaborative machine tool maintenance planning system based on content management technologies Int. J. Adv. Manuf. Technol., 94 (2018), pp. 1639-1653
- [94] W. Luo, T. Hu, Y. Ye, C. Zhang, Y. Wei A hybrid predictive maintenance approach for CNC machine tool driven by Digital Twin Robot Comput. Integr. Manuf., 65 (2020), Article 101974
- [95] J. Moore, J. Stammers, J. Dominguez-Caballero The application of machine learning to sensor signals for machine tool and process health assessment Proc. Inst. Mech. Eng. Part B J. Eng. Manuf., 235 (2021), pp. 1543-1557
- [96] T. Mohanraj, J. Yerchuru, H. Krishnan, R.N. Aravind, R. Yameni Development of tool condition monitoring system in end milling process using wavelet features and Hoelder’s exponent with machine learning algorithms Measurement, 173 (2021), Article 108671
- [97] A.D. Patange, R. Jegadeeshwaran Review on tool condition classification in milling: a machine learning approach Mater. Today: Proc., 46 (2021), pp. 1106-1115
- [98] D. Goyal, C. Mongia, S. Sehgal Applications of digital signal processing in monitoring machining processes and rotary components: a review IEEE Sens. J., 21 (2021), pp. 8780-8804
- [99] K. Zhu, Y. Zhang A cyber-physical production system framework of smart CNC machining monitoring system IEEE/ASME Trans. Mechatron., 23 (2018), pp. 2579-2586
- [100] C. Ratnam, K.A. Vikram, B. Ben, B. Murthy Process monitoring and effects of process parameters on responses in turn-milling operations based on SN ratio and ANOVA Measurement, 94 (2016), pp. 221-232
- [101] S. Wang, S. To, C.F. Cheung Effect of workpiece material on surface roughness in ultraprecision raster milling Mater. Manuf. Process., 27 (2012), pp. 1022-1028
- [102] S. Jovic, O. Anicic, M. Jovanovic Adaptive neuro-fuzzy fusion of multi-sensor data for monitoring of CNC machining Sensor Rev. (2017)
- [103] S. Liu, S. Lu, J. Li, X. Sun, Y. Lu, J. Bao Machining process-oriented monitoring method based on digital twin via augmented reality Int. J. Adv. Manuf. Technol., 113 (2021), pp. 3491-3508
- [104] I. Daniyan, I. Tlhabadira, O. Daramola, K. Mpofu Design and optimization of machining parameters for effective AISI P20 removal rate during milling operation Procedia CIRP, 84 (2019), pp. 861-867
- [105] K. Zacharia, P. Krishnakumar Chatter prediction in high speed machining of titanium alloy (Ti-6Al-4V) using machine learning techniques Mater. Today: Proc., 24 (2020), pp. 350-358
- [106] A. Gouarir, G. Martínez-Arellano, G. Terrazas, P. Benardos, S. Ratchev In-process tool wear prediction system based on machine learning techniques and force analysis Procedia CIRP, 77 (2018), pp. 501-504
- [107] E. Hazir, T. Ozcan Response surface methodology integrated with desirability function and genetic algorithm approach for the optimization of CNC machining parameters Arab. J. Sci. Eng., 44 (2019), pp. 2795-2809
- [108] K. Martinsen, J. Downey, I. Baturynska Human-machine interface for artificial neural network based machine tool process monitoring Procedia CIRP, 41 (2016), pp. 933-938
- [109] I. Asiltürk, S. Neşeli, M.A. Ince Optimisation of parameters affecting surface roughness of Co28Cr6Mo medical material during CNC lathe machining by using the Taguchi and RSM methods Measurement, 78 (2016), pp. 120-128
- [110] C.-.H. Chen, S.-.Y. Jeng, C.-.J. Lin Prediction and analysis of the surface roughness in CNC end milling using neural networks Appl. Sci., 12 (2021), p. 393
- [111] N.E. Sizemore, M.L. Nogueira, N.P. Greis, M.A. Davies Application of machine learning to the prediction of surface roughness in diamond machining Procedia Manuf., 48 (2020), pp. 1029-1040
- [112] W. Zhang Surface roughness prediction with machine learning J. Phys.: Conf. Ser. (2021), Article 012040 IOP Publishing
- [113] H.-.W. Chiu, C.-.H. Lee Prediction of machining accuracy and surface quality for CNC machine tools using data driven approach Adv. Eng. Software, 114 (2017), pp. 246-257
- [114] W.-.J. Lin, S.-.H. Lo, H.-.T. Young, C.-.L. Hung Evaluation of deep learning neural networks for surface roughness prediction using vibration signal analysis Appl. Sci., 9 (2019), p. 1462
- [115] D.Y. Pimenov, A. Bustillo, T. Mikolajczyk Artificial intelligence for automatic prediction of required surface roughness by monitoring wear on face mill teeth J. Intell. Manuf., 29 (2018), pp. 1045-1061
- [116] N. Fang, P.S. Pai, N. Edwards Neural network modeling and prediction of surface roughness in machining aluminum alloys J. Comput. Commun., 4 (2016), pp. 1-9
- [117] A.N. Balasubramanian, N. Yadav, A. Tiwari Analysis of cutting forces in helical ball end milling process using machine learning Mater. Today: Proc., 46 (2021), pp. 9275-9280
- [118] W. Gao, H. Haitjema, F. Fang, R. Leach, C. Cheung, E. Savio, J.-.M. Linares On-machine and in-process surface metrology for precision manufacturing CIRP Ann., 68 (2019), pp. 843-866
- [119] H.-.C. Möhring, S. Eschelbacher, P. Georgi Machine learning approaches for real-time monitoring and evaluation of surface roughness using a sensory milling tool Procedia CIRP, 102 (2021), pp. 264-269
- [120] P. Wang, Z. Liu, R.X. Gao, Y. Guo Heterogeneous data-driven hybrid machine learning for tool condition prognosis CIRP Ann., 68 (2019), pp. 455-458
- [121] X. Xu, J. Wang, B. Zhong, W. Ming, M. Chen Deep learning-based tool wear prediction and its application for machining process using multi-scale feature fusion and channel attention mechanism Measurement, 177 (2021), Article 109254
- [122] Y. Chen, R. Sun, Y. Gao, J. Leopold A nested-ANN prediction model for surface roughness considering the effects of cutting forces and tool vibrations Measurement, 98 (2017), pp. 25-34
- [123] M.K.M. Shapi, N.A. Ramli, L.J. Awalin Energy consumption prediction by using machine learning for smart building: case study in Malaysia Dev. Built Environ., 5 (2021), Article 100037
- [124] J. Pan, C. Li, Y. Tang, W. Li, X. Li Energy consumption prediction of a CNC machining process with incomplete data IEEE/CAA J. Automat. Sin., 8 (2021), pp. 987-1000
- [125] A. Mosavi, A. Bahmani, Energy consumption prediction using machine learning; a review, (2019).
- [126] C. Chen, Y. Liu, M. Kumar, J. Qin, Y. Ren Energy consumption modelling using deep learning embedded semi-supervised learning Comput. Ind. Eng., 135 (2019), pp. 757-765
- [127] P.W. Khan, Y. Kim, Y.-.C. Byun, S.-.J. Lee Influencing factors evaluation of machine learning-based energy consumption prediction Energies, 14 (2021), p. 7167
- [128] J. Cao, X. Xia, L. Wang, Z. Zhang, X. Liu A novel CNC milling energy consumption prediction method based on program parsing and parallel neural network Sustainability, 13 (2021), p. 13918
- [129] Y. He, P. Wu, Y. Li, Y. Wang, F. Tao, Y. Wang A generic energy prediction model of machine tools using deep learning algorithms Appl. Energy, 275 (2020), Article 115402
- [130] Z. Liu, Y. Guo A hybrid approach to integrate machine learning and process mechanics for the prediction of specific cutting energy CIRP Ann., 67 (2018), pp. 57-60
- [131] V. Vishnu, K.G. Varghese, B. Gurumoorthy Energy prediction in process planning of five-axis machining by data-driven modelling Procedia CIRP, 93 (2020), pp. 862-867
- [132] W.H. Choi, J. Kim, J.Y. Lee Development of fault diagnosis models based on predicting energy consumption of a machine tool spindle Procedia Manuf., 51 (2020), pp. 353-358
- [133] L. Li, C. Li, Y. Tang, L. Li An integrated approach of process planning and cutting parameter optimization for energy-aware CNC machining J. Clean. Prod., 162 (2017), pp. 458-473
- [134] S. Jia, S. Wang, J. Lv, W. Cai, N. Zhang, Z. Zhang, S. Bai Multi-objective optimization of CNC turning process parameters considering transient-steady state energy consumption Sustainability, 13 (2021), p. 13803
CNC Machining Industry: new & important trend by CapableMaching is licensed under CC BY-NC 4.0